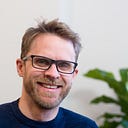

Discover more from AI Impacts blog
Product safety is a poor model for AI governance
Rick Korzekwa, February 1, 2023
Note: This post is intended to be accessible to readers with relatively little background in AI safety. People with a firm understanding of AI safety may find it too basic, though they may be interested in knowing which kinds of policies I have been encountering or telling me if I've gotten something wrong.
I have recently encountered many proposals for policies and regulations intended to reduce risk from advanced AI. These proposals are highly varied and most of them seem to be well-thought-out, at least on some axes. But many of them fail to confront the technical and strategic realities of safely creating powerful AI, and they often fail in similar ways. In this post, I will describe a common type of proposal1 and give a basic overview of the reasons why it is inadequate. I will not address any issues related to the feasibility of implementing such a policy.
Caveats
It is likely that I have misunderstood some proposals and that they are already addressing my concerns. Moreover, I do not recommend dismissing a proposal because it pattern-matches to product safety on the surface level.
These approaches may be good when combined with others. It is plausible to me that an effective and comprehensive approach to governing AI will include product-safety-like regulations, especially early in the process when we’re still learning and setting the groundwork for more mature policies.
The product safety model of AI governance
A common AI policy structure, which I will call the ‘product safety model of AI governance’2, seems to be built on the assumption that, while the processes involved in creating powerful AI may need to be regulated, the harm from a failure to ensure safety occurs predominantly when the AI has been deployed into the world. Under this model, the primary feedback loop for ensuring safety is based on the behavior of the model after it has been built. The product is developed, evaluated for safety, and either sent back for more development or allowed to be deployed, depending on the evaluation. For a typical example, here is a diagram from a US Department of Defense report on responsible AI:3
The system in this diagram is not formally evaluated for safety or performance until after “Acquisition/Development”.4
I do not find it surprising that this model is so common. Most of the time when we are concerned about risk from technology, we are worried about what happens when the technology has been released into the world. A faulty brake line on a car is not much of a concern to the public until the car is on public roads, and the facebook feed algorithm cannot be a threat to society until it is used to control what large numbers of people see on their screens. I also think it is reasonable to start with regulations that are already well-understood, and work from there. But this model, on its own, is inadequate for AI, for reasons I will explain in the next section.
This model fails when applied to advanced AI
This approach cannot be relied on to prevent the major risks associated with advanced AI. I will give two reasons for this, though there may be others.
Safety cannot (yet) be evaluated reliably in a fully-developed AI
A fundamental difficulty of ensuring that AI will behave as intended is that examining an AI or observing its behavior during development and testing is not a reliable indicator of how it will behave once deployed. This is a difficult, unsolved problem that resists simple solutions.5 I will not explain this problem in detail, but I will list some aspects of it here, along with real-world examples, and you can read more about it elsewhere.67
AI may learn approaches to problems or, if sufficiently advanced, acquire goals that result in behavior that seems to work in training but will fail in deployment.
A system trained to classify images performed extremely well, but only because it had learned to detect images based on their storage location.8
A simulated robot was trained using human feedback. It was supposed to grasp an object, but instead it learned to place its hand between the object and the camera, giving the appearance of grasping it.9
AI can find solutions to problems that cannot be reliably evaluated by humans.
An evolutionary algorithm for designing circuits on a real circuit board created a circuit that produced the desired output, but the researchers were unable to determine how it worked.10
AI with goals that are at odds with human interests will have an incentive to avoid behavior that reveals its goals.
Creatures undergoing simulated evolution learned to identify when they were in an evaluation environment and when they were in the “real” environment, and used this to hide their unwanted behavior.11
Unsafe AI can cause substantial harm before deployment
Unlike most technologies, AI can pose a serious risk even during development and testing. As AI capabilities approach and surpass human capabilities, the capacity for AI to cause harm will increase and it will become more difficult to ensure that AI is unable to cause harm outside its development and testing environment. I will not go into lots of detail on this, but I will outline the basic reasons for concern.
AI is likely to seek influence outside its intended domain
Whether a given AI system will attempt to gain influence outside the environment in which it is trained and tested is difficult to determine, but we have ample reason to be cautious. Learning to create and execute plans toward a particular goal is something we should expect by default within a broad range of AI applications as capabilities increase12, and for a system to do well on real-world tasks, it will need to make use of information about the wider world. This is something that current language models are already doing on their own.13 There are also examples of AI finding vulnerabilities that allow it to manipulate things it was not intended to have access to, such as a game-playing AI that exploited a glitch in the game to increase its score directly.14
It is difficult to constrain advanced AI to a particular domain
AI with access to the Internet may create copies of itself, gain access to influential systems such as critical infrastructure, or manipulate people into taking actions that are harmful or increase its influence. AI without direct access to the outside world may be able to gain access by manipulating people involved in its development or by exploiting hardware vulnerabilities.15
Executing such an escape from a datacenter may not require very high capability. Surprisingly simple social engineering techniques16 can be successful at getting a person to do something that is not in their interest. Malware that can propagate between computers and control them has existed for a long time17 and does not require the software to have any high-level decision making capability at all.
Importantly, detecting such escape attempts may be very difficult. Almost any AI will have a strong incentive not to be observed taking dangerous, unintended actions, and current systems sometimes find unintended solutions that are invisible to humans.18 Additionally, AI in training is subject to the evaluation difficulties explained in the first section.
AI breakout could be catastrophic
Once AI has gained influence outside its intended domain, it could cause immense harm. How likely this is to happen and how much harm it may cause is a big topic19 that I will not try to cover here, but there are a few things worth pointing out in this context:
Most of the arguments around catastrophic AI risk are agnostic to whether AI gains influence before or after deployment.
The basic premise behind catastrophic AI risk is not just that it may use whatever influence it is granted to cause harm, but that it will seek additional influence. This is at odds with the basic idea behind the product safety model, which is that we prevent harm by only granting influence to AI that has been verified as safe.
The overall stakes are much higher than those associated with things we normally apply the product safety model to, and unlike most risks from technology, the costs are wide reaching and almost entirely external.
Additional comments
I am reluctant to offer alternative models, in part because I want to keep the scope of this post narrow, but also because I'm not sure which approaches are viable. But I can at least provide some guiding principles:
Policies intended to mitigate risk from advanced AI must recognize that verifying the safety of highly capable AI systems is an unsolved problem that may remain unsolved for the foreseeable future.
These policies must also recognize that public risk from AI begins during development, not at the time of deployment.
More broadly, it is important to view AI risk as distinct from most other technologies. It is poorly understood, difficult to control in a reliable and verifiable way, and may become very dangerous before it becomes well understood.
Finally, I think it is important to emphasize that, while it is tempting to wait until we see the warning signs that AI is becoming dangerous before enacting such policies, capabilities are advancing rapidly and AI may acquire the ability to seek power and conceal its actions in a sophisticated way with relatively little warning.
Acknowledgements
Thanks to Harlan Stewart for giving feedback and helping with citations, Irina Gueorguiev for productive conversations on this topic, and Aysja Johnson, Jeffrey Heninger, and Zach Stein-Perlman for feedback on an earlier draft. All mistakes are my own.
They seem common to me, based mainly on conversations, posts, and presentations I've seen within the AI governance field. It is possible these kinds of proposals are rare, but memorable to me.
I am not at all an expert on real product safety regulations. This is meant mainly as a useful comparison, not a criticism of product safety regulation in general.
DoD Responsible AI Working Council (U.S.). U.S. Department of Defense Responsible Artificial Intelligence Strategy and Implementation Pathway. 2022.
https://www.ai.mil/docs/RAI_Strategy_and_Implementation_Pathway_6-21-22.pdf
The text of the article does not rule out intervention in development or basic research, but it does not seem to consider this a crucial aspect of ensuring safety.
For an accessible and engaging introduction: Christian B. The Alignment Problem: Machine Learning and Human Values. New York, NY: W.W. Norton & Company; 2020.
Amodei D, Olah C, Steinhardt J et al. Concrete Problems in AI Safety. arXiv. Preprint posted online June 21, 2016. https://arxiv.org/pdf/1606.06565.pdf
Ngo R. The alignment problem from a deep learning perspective. arXiv. Preprint posted online August 30, 2022. https://arxiv.org/abs/2209.00626
From Hacker News user api:
“To give a specific example: I once wrote an objective function to train an evolving system to classify images, a simple machine learning test. After running it for only an hour or so, the system's performance seemed spectacular, like way up in the 90'th percentile. This made me suspicious. The programs that had evolved did not seem complex enough, and past experiments had shown that it should take a lot longer to get something that showed reasonable performance.
After a lot of analysis I figured out what it was.
I was pulling test images from two different databases. One database had higher latency than the other. The bugs had evolved a timing loop to measure how long it took them to get their data (they were multi-threaded) and were basically executing a side-channel attack against the training supervisor.”
https://news.ycombinator.com/item?id=6269114
“in some domains our system can result in agents adopting policies that trick the evaluators. For example, a robot which was supposed to grasp items instead positioned its manipulator in between the camera and the object so that it only appeared to be grasping it, as shown below.”
OpenAI. “Learning from Human Preferences,” June 13, 2017. https://openai.com/blog/deep-reinforcement-learning-from-human-preferences/.
“It has proved difficult to clarify exactly how these circuits work. Probing a typical one with an oscilloscope has shown that it does not use beat frequencies to achieve the target frequency. If the transistors are swapped for nominally identical ones, then the output frequency changes by as much as 30%. A simulation was created that incorporated all the parasitic capacitance expected to exist within the physical circuit, but the simulated circuits failed to oscillate. The programmable switches almost certainly play an important role in the behaviour of the circuit and it is only possible to probe their input and output connections and not the circuitry in which they are embedded.”
Bird, Jon, and Paul Layzell. "The evolved radio and its implications for modelling the evolution of novel sensors." In Proceedings of the 2002 Congress on Evolutionary Computation. CEC'02 (Cat. No. 02TH8600), vol. 2, pp. 1836-1841. IEEE, 2002.
“[Charles Ofria, the researcher] tried to disable mutations that improved an organism’s replication rate (i.e. its fitness). He configured the system to pause every time a mutation occurred, and then measured the mutant’s replication rate in an isolated test environment. If the mutant replicated faster than its parent, then the system eliminated the mutant; otherwise, the mutant would remain in the population…However, while replication rates at first remained constant, they later unexpectedly started again rising. After a period of surprise and confusion, Ofria discovered that he was not changing the inputs provided to the organisms in the isolated test environment. The organisms had evolved to recognize those inputs and halt their replication”
Lehman, Joel, Jeff Clune, Dusan Misevic, Christoph Adami, Lee Altenberg, Julie Beaulieu, Peter J. Bentley et al. "The surprising creativity of digital evolution: A collection of anecdotes from the evolutionary computation and artificial life research communities." arXiv preprint arXiv:1803.03453 (2018).
“Many real-world environments have symmetries which produce power-seeking incentives. In particular, optimal policies tend to seek power when the agent can be shut down or destroyed. Seeking control over the environment will often involve resisting shutdown, and perhaps monopolizing resources.”
Turner AM, Smith L, Shah R et al. Optimal Policies Tend to Seek Power. arXiv. Preprint posted online December 3, 2021. https://arxiv.org/abs/1912.01683
“As one early example, when Degrave [2022] prompted OpenAI’s ChatGPT language model to output the source code at its own URL, it hallucinated code which called a large language model with similar properties as itself. This suggests that the ChatGPT training data contained enough information about OpenAI for ChatGPT to infer some plausible properties of an OpenAI-hosted URL. More generally, large language models trained on internet text can extensively recount information about deep learning, neural networks, and the real-world contexts in which those networks are typically deployed; and can be fine-tuned to recount details about themselves specifically [OpenAI, 2022a]. We should expect future models to learn to consistently use this information when choosing actions, because that would contribute to higher reward on many training tasks.”
https://arxiv.org/abs/2209.00626
“In the second interesting solution (https://www. youtube.com/watch?v=meE5aaRJ0Zs), the agent discovers an in-game bug. First, it completes the first level and then starts to jump from platform to platform in what seems to be a random manner. For a reason unknown to us, the game does not advance to the second round but the platforms start to blink and the agent quickly gains a huge amount of points (close to 1 million for our episode time limit)”
Chrabaszcz P, Loshchilov I, Hutter F. Back to Basics: Benchmarking Canonical Evolution Strategies for Playing Atari. arXiv. Preprint posted online February 24, 2018. https://arxiv.org/abs/1802.08842
“Academics from an Israeli university have published new research today detailing a technique to convert a RAM card into an impromptu wireless emitter and transmit sensitive data from inside a non-networked air-gapped computer that has no Wi-Fi card.”
Cimpanu C. Academics Turn Ram into wi-fi cards to steal data from air-gapped systems. ZDNET. https://www.zdnet.com/article/academics-turn-ram-into-wifi-cards-to-steal-data-from-air-gapped-systems. Published December 15, 2020. Accessed October 3, 2022.
“Phishing emails and text messages often tell a story to trick you into clicking on a link or opening an attachment. You might get an unexpected email or text message that looks like it’s from a company you know or trust, like a bank or a credit card or utility company. Or maybe it’s from an online payment website or app.”
How to recognize and avoid phishing scams. Federal Trade Commission Consumer Advice. https://consumer.ftc.gov/articles/how-recognize-and-avoid-phishing-scams. Published September 2022. Accessed October 3, 2022.
“At around 8:30 p.m. on November 2, 1988, a maliciously clever program was unleashed on the Internet from a computer at the Massachusetts Institute of Technology (MIT).This cyber worm was soon propagating at remarkable speed and grinding computers to a halt. ‘We are currently under attack,’ wrote a concerned student at the University of California, Berkeley in an email later that night. Within 24 hours, an estimated 6,000 of the approximately 60,000 computers that were then connected to the Internet had been hit. Computer worms, unlike viruses, do not need a software host but can exist and propagate on their own.”
The Morris Worm. FBI.gov. https://www.fbi.gov/news/stories/morris-worm-30-years-since-first-major-attack-on-internet-110218. Published November 2, 2018. Accessed October 3, 2022.
“In a series of experiments, we demonstrate an intriguing property of the model: CycleGAN learns to ‘hide’ information about a source image into the images it generates in a nearly imperceptible, highfrequency signal.”
Chu C, Zhmoginov A, Sandler M. CycleGAN, a Master of Steganography. arXiv. Preprint posted online December 16, 2017. https://arxiv.org/abs/1712.02950
List of sources arguing for existential risk from AI. AI Impacts. https://aiimpacts.org/list-of-sources-arguing-for-existential-risk-from-ai/. Published August 6, 2022. Accessed October 3, 2022.
Subscribe to AI Impacts blog
A blog from AI Impacts about the future of artificial intelligence